Understanding Image Annotation for Machine Learning
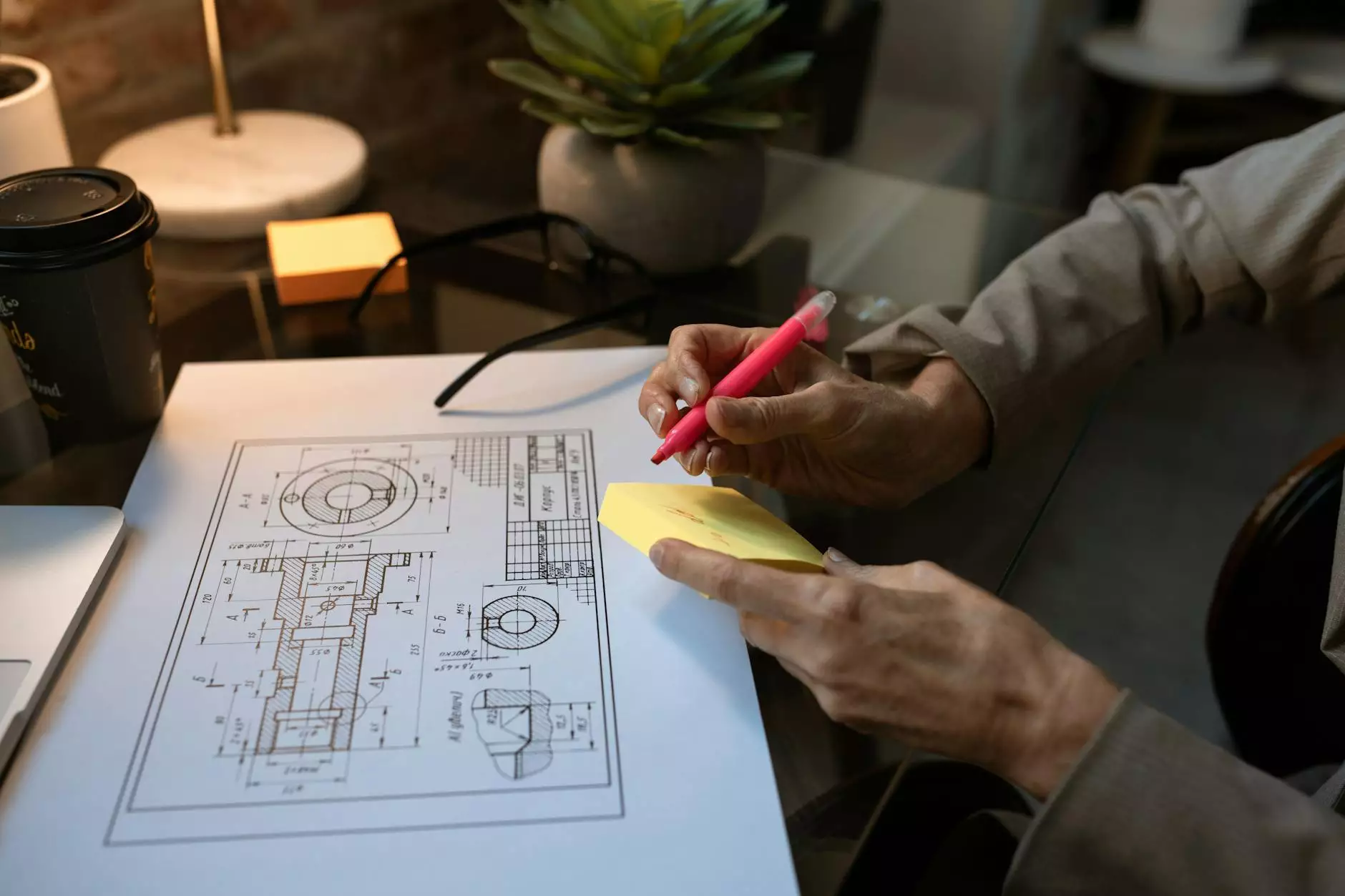
In today's digital landscape, the significance of image annotation for machine learning cannot be overstated. As machine learning algorithms become increasingly advanced, the demand for quality data has surged. This article will explore the intricacies of image annotation, its applications, techniques, and why it is essential for developing robust machine learning models.
The Importance of Image Annotation in Machine Learning
Image annotation refers to the process of labeling images with metadata that helps in training machine learning models. In a world where visual data dominates, the ability to accurately identify and categorize images is crucial. The primary goal of image annotation is to make machine learning systems understand images the way humans do.
Why should businesses prioritize image annotation for machine learning? Here are several compelling reasons:
- Enhanced Accuracy: Accurate data leads to better model predictions.
- Increased Efficiency: Well-annotated datasets allow faster model training.
- Scalability: Annotated datasets can easily be scaled to accommodate larger machine learning projects.
- Cost-effectiveness: High-quality annotated images reduce the need for extensive trial and error in training.
Types of Image Annotation
There are several image annotation techniques used in machine learning, each serving different purposes depending on the requirements of the project. Below are the most common types:
1. Bounding Box Annotation
This technique involves drawing a box around the object of interest in an image. It is commonly used in object detection tasks.
2. Polygon Annotation
For more complex shapes, polygon annotation is used, which allows for more precise labeling of objects that are not rectangular.
3. Semantic Segmentation
This involves classifying each pixel in the image to a specific class label. This is vital for tasks requiring an understanding of shapes and boundaries.
4. Instance Segmentation
This method combines object detection and semantic segmentation, allowing models to differentiate between individual objects of the same class.
5. Keypoint Annotation
Keypoint annotation captures specific points on objects, making it invaluable in tasks such as facial recognition and pose estimation.
Challenges in Image Annotation
Despite its advantages, image annotation for machine learning comes with its unique set of challenges:
- Time-Consuming: Annotating images can be a lengthy process, particularly for massive datasets.
- Subjectivity: Different annotators may interpret images differently, leading to inconsistencies.
- Cost: Hiring skilled annotators can be expensive, affecting project budgets.
- Data Privacy: Handling sensitive images poses legal risks related to privacy regulations.
To Overcome Challenges: Choosing the Right Data Annotation Tool
Selecting the right data annotation tool is essential for ensuring quality and efficiency in the annotation process. Here are some features to consider:
1. User-Friendly Interface
The tool should have an intuitive interface that makes it easy for annotators to label images efficiently.
2. Automation Capabilities
Tools that incorporate AI-driven automation can significantly reduce the time required for annotation.
3. Versatility
Versatile tools that support various annotation techniques (bounding boxes, polygons, etc.) are beneficial for diverse projects.
4. Quality Control Mechanisms
Integrated quality control features can help monitor annotation accuracy and maintain consistency across the dataset.
5. Scalability
A good data annotation platform should easily scale as project demands grow.
The Role of KeyLabs.ai in Image Annotation
At KeyLabs.ai, we understand the critical role that image annotation for machine learning plays in developing AI models. Our state-of-the-art data annotation platform is designed to provide high-quality annotations that simplify and accelerate the machine learning process.
Why Choose KeyLabs.ai?
- Expert Annotation Team: Our skilled annotators ensure that every image is accurately labeled, providing reliable data for training.
- Advanced Technology: Utilizing cutting-edge machine learning techniques, we offer tools that enhance productivity and efficiency.
- Custom Solutions: We tailor our services to meet the unique needs of each client, ensuring a personalized experience.
- Robust Security: Commitment to data privacy and security is paramount, allowing clients to trust us with sensitive information.
Future Trends in Image Annotation for Machine Learning
As machine learning continues to evolve, so too does image annotation. Here are some exciting trends to watch:
1. Augmented Reality (AR) and Virtual Reality (VR)
AR and VR technologies are gaining traction, demanding new methods of image annotation to enhance these immersive environments.
2. Real-Time Annotation
Developments in AI are paving the way for real-time image annotation, which could revolutionize industries like healthcare and autonomous driving.
3. Crowdsourced Annotation
Crowdsourcing is becoming an increasingly popular method for gathering diverse annotations, leading to richer datasets and faster delivery times.
4. AI-Assisted Annotation
AI algorithms will play a significant role in pre-annotating images, reducing manual effort and improving the quality of annotations.
5. Enhanced Collaboration Tools
Future platforms will likely feature better collaboration tools that facilitate communication between teams and annotators, promoting efficiency and accuracy.
Conclusion
The landscape of image annotation for machine learning is continuously evolving, shaped by technological advancements and the growing need for high-quality annotated data. By partnering with leaders in the field, such as KeyLabs.ai, businesses can leverage premium annotation services to enhance their machine learning initiatives.
The benefits of adopting an effective image annotation strategy are clear: improved model accuracy, reduced project timelines, and ultimately, a competitive edge in the rapidly changing tech landscape. As we look to the future, staying informed about trends and innovations in image annotation will be vital for any business aiming to stay ahead in the machine learning game.